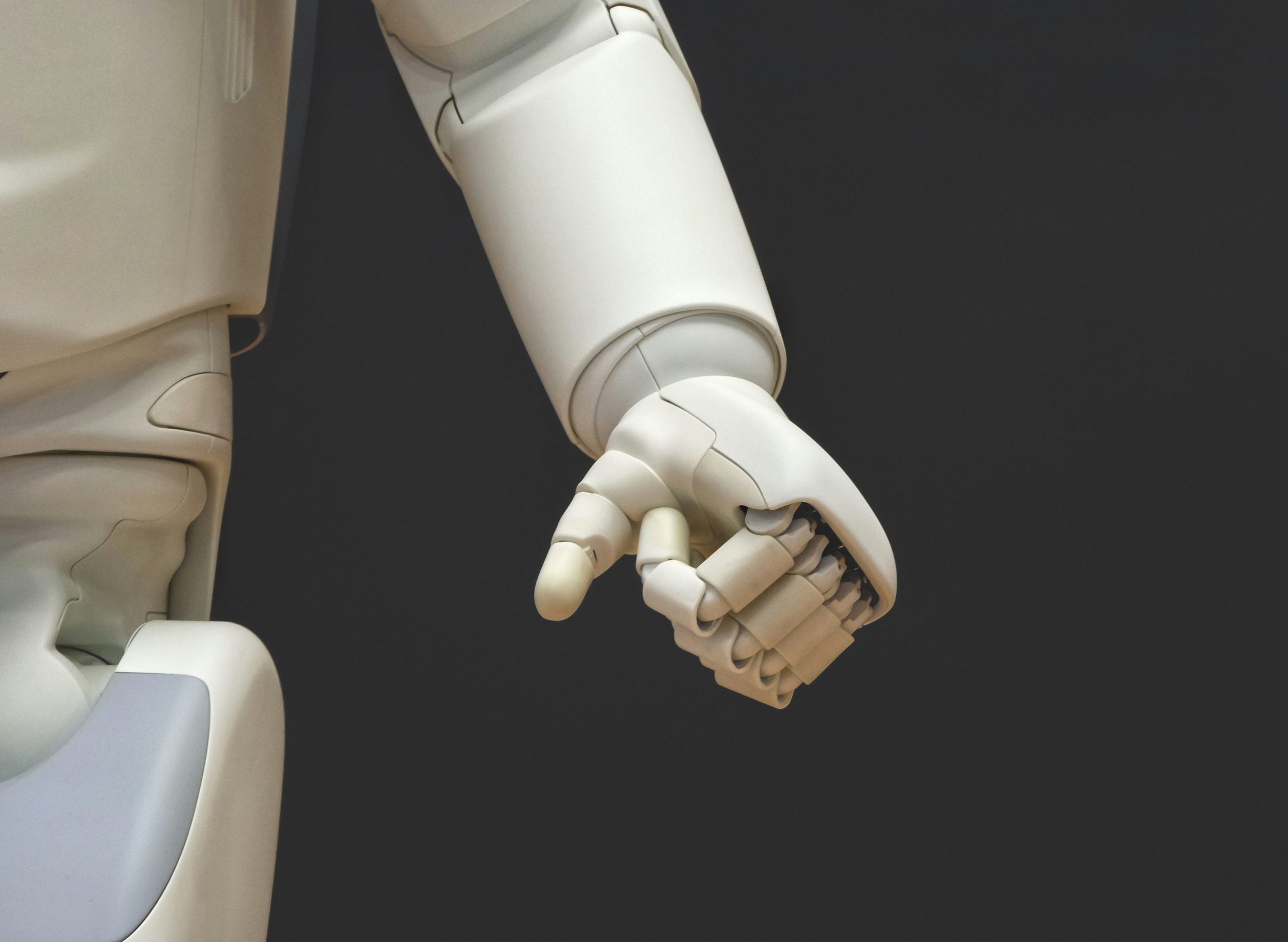
Anatomy of a Fraud Bot Attack
We’re hearing a lot about genAI lately, and especially how it’s making automated fraud a lot easier—from bot development to deployment at scale.
Because NeuroID behavioral analytics was built in part specifically for bot detection, we’ve been following these changing attack tactics for some time. Our own attack data shows that new hybrid bot-human attack strategies are becoming more powerful and that genAI is supercharging bot development, which in turn enables fraud attacks at an unprecedented speed and scale.
At the same time, we know fraud bot attack styles don’t sunset as the next generation rises. Fraudsters deploy different bots in different ways for different purposes. We tell our customers that the best approach is a fraud stack ready for all past, present, and future fraud bot generations.
The trick is balancing readiness against data-overwhelm. Advanced technologies, such as behavioral analytics, are critical for detecting next-generation bots. But at the same time, device and network intelligence remain essential to finding the first- and second-generation bots that aren’t going away any time soon. After all, fraudsters will always choose the easiest path forward—why use a fourth-gen bot when a first-gen bot will do?
Your first line of defense is knowledge, and understanding the signs of different bot attack styles can help. When you know the signs and the best points to detect them within your fraud stack, you can better prepare your defenses. Here are 3 common attack methodologies we’ve seen patterns within our customer database, broken down by style, strategy, and success level, and what you can look for in your own attack data.
Bot Attack Target: Consumer Financial Manager’s Digital Application Process
The Attack: Our behavioral analytics picked up a bot surge across a consumer financial manager’s digital application process. We noticed a pattern of spikes: each one started with a human fraudster testing for weakness, followed by a probe of bots, leading finally to a flood of bots that lasted for two weeks.
The Indicators: The set-up pattern of humans ahead of bots became clear, as the fraudster-pair worked in tandem toward the huge spike onslaught.
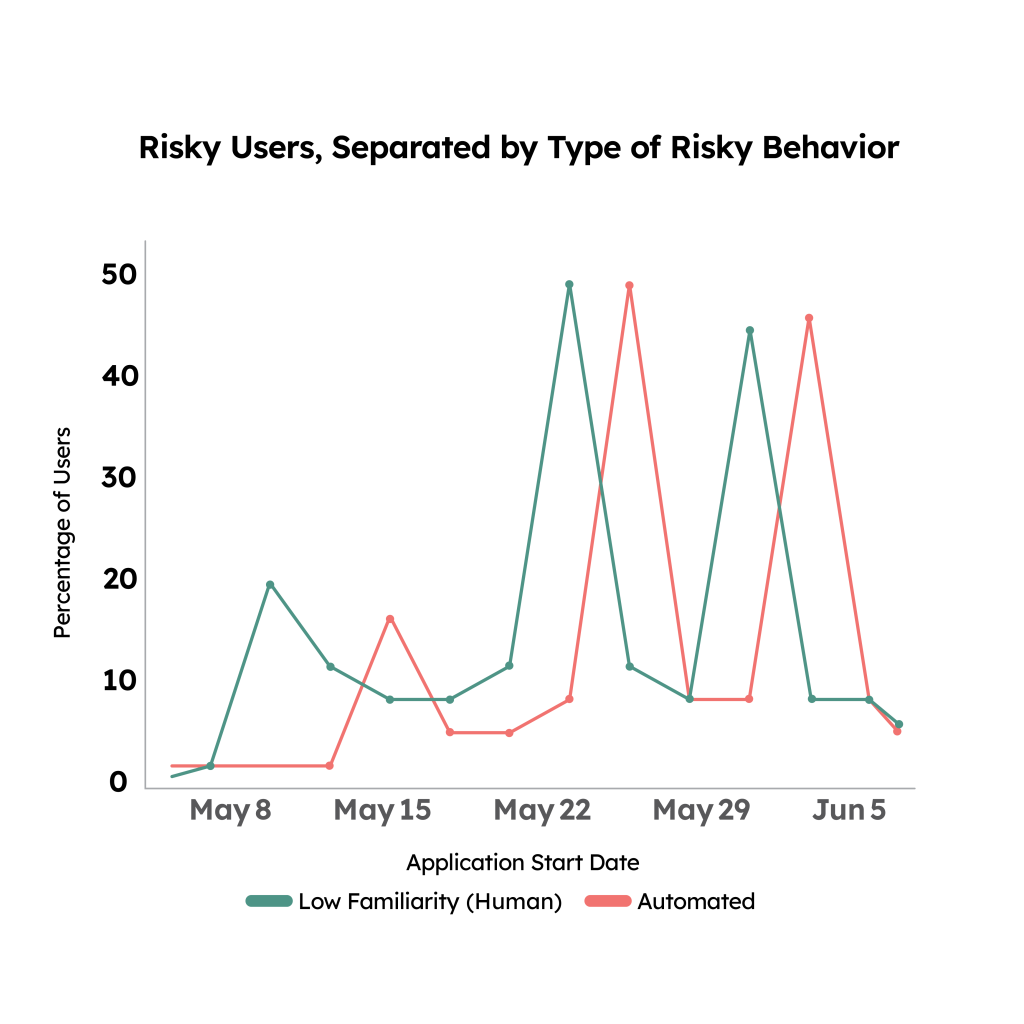
The Result: Was it a bot script that fraud tools weren’t equipped to mitigate? Was it human intervention combined with bot speed? The end result was the same: fraudsters were making it past traditional verifications.
The Takeaway: During that week, 50% of all this consumer financial manager’s traffic were bots, nearly triple the bot attack volume compared to the company’s average baseline. Not only that, but as they switched step-ups and controls to adjust one point of entry, the attacks seamlessly changed to another entry-point. These fraudsters had done their homework (likely the human probe patterns we saw hit with the earlier spikes) and knew which vectors would close and open.
In a post-analysis, it was clear that without behavioral analytics, a fraud team would have had to keep up a high-stakes game of whack-a-mole, trying to thwart bots as they switched to a new entry point. Without the behavioral patterns to look for, they also wouldn’t have been able to follow trends to find fraudsters who made it past traditional controls.
Luckily, with NeuroID, they stopped these bots no matter how many entry-points they switched to: behavioral traits gave them away instantly.
Read the full case study here.
Bot Attack Target: Payment Processor’s Sign-Up Page
The Attack: We detected a small surge in risky human users followed by a larger spike in bot activity on a payment processor’s sign-up page. The bots weren’t necessarily attempting to complete the sign-up, but rather testing the limits of the payment processor’s fraud checks (we call this “probing” behavior).
The Indicators: Lurking under a 2X increase in overall users was a surge in both risky human and bot activity. The payment processor first experienced a 7 percentage point jump in human risky activity, then was struck by a 31% spike in automated activity.
The Result: By manually probing before sending in bots to learn more, fraudsters were able to discover what did and didn’t trigger a step-up in the payment processor’s sign-up process. If the payment processor didn’t have behavioral analytics to reveal the spikes in fraudulent activity, fraudsters would’ve been able to turn their learnings into a large-scale, devastating attack.
The Takeaway: As fraudsters poke and prod fraud checks to find step up triggers, small spikes in risky human and automated activity can be a precursor to larger attacks. Behavioral analytics provides visibility into these spikes, giving businesses the knowledge to see and stop a forthcoming attack. This is a prime example of how traditional, patient fraud rings can cause havoc even without the high-sophistication of genAI. If you’re not watching for the right signs, it’s easy for them to slip in.
Bot Attack Target: Top Bank’s Onboarding Volume
The Attack: Fraudsters deployed thousands of next-generation, genAI-powered bots on a bank’s onboarding flow. These fraud bots were sophisticated enough to appear human and didn’t trip any of the bank’s multi-layered detection systems.
The Indicators: We saw high verified fraud volume, but few fraudulent accounts were being caught at onboarding. There was clearly a new, advanced type of fraud bot being used in this attack that was extremely difficult to differentiate from a normal human.
The Result: The next-generation bots weren’t detected by any other tool in the bank’s fraud stack. NeuroID investigated the attack and identified the sophisticated bots leading the attack, allowing the bank to discover 20K bots that were previously undetected and likely lying in wait as dormant fraud.
The Takeaway: Modern bots require modern solutions. NeuroID’s fraud bot detection signal is designed to spot the nuances that can reveal a next-generation bot (which have been specifically trained to evade behavioral analytics).
Fourth-generation bots have essentially reverse-engineered fraud stacks through genAI and evolved to be much more human-like in their behavior (since behavioral analytics was one of the best defenses against earlier bots).
But there are still always discrete signs of a bot or script, and our models have been trained to detect them. NeuroID behavioral analytics can uncover the wide range of bot generations, from simple scripts to sophisticated behavior-based spoofs.
Want to learn more? Download our report, Fighting the Future of Fraud: Understanding and Combating Next-Gen Bots for a full look at bots’ evolution and NeuroID’s bot detection capabilities.