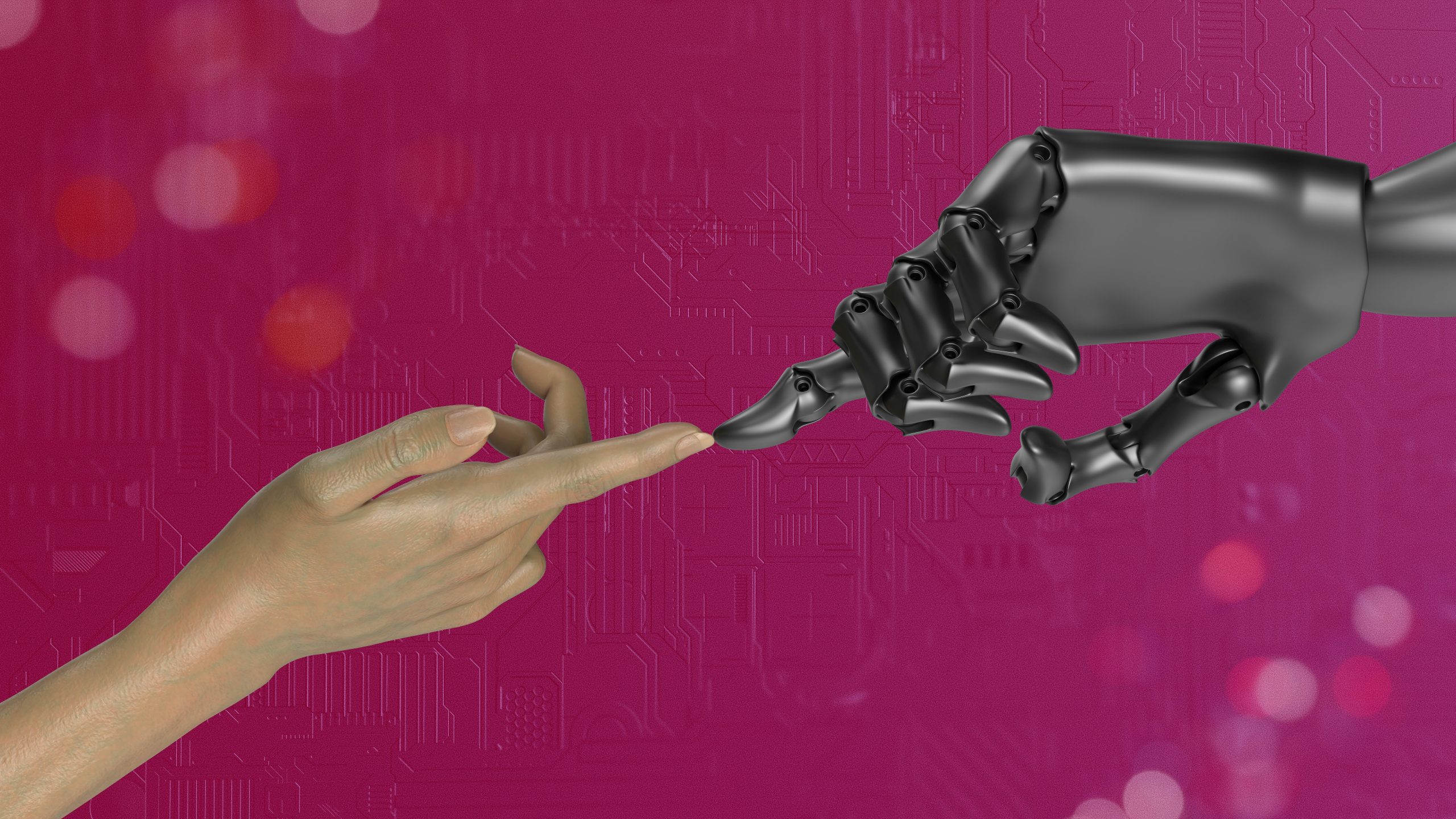
Have Bots Finally Beat Behavior?
From the first-generation of bots that deployed simple, basic scripting through to the third-generation bots that operate through full-fledged browsers, these fraud automatons have consistently had a huge weakness: They weren’t human. And behavioral analytics could tell, able to identify programmatic sequences in their taps, swipes, clicks, and other nuances that gave them away.
Until now.
This power to detect bots that other solutions missed has put a huge target on behavioral analytics’ back. What’s NeuroID (an industry-leading behavioral analytics company) supposed to do when bots have evolved SPECIFICALLY to defeat behavioral analytics?
How Did Bots Get This Advanced?
Every digital financial services’ fraud team has a somewhat standard set of fraud tools, step-ups, and checks to defeat fraudsters in one way or another. One of the reasons that generative AI (genAI) is so powerful as a fraud tool is because it was built to analyze large, diverse datasets, identify patterns and structures, and then use those patterns to generate solutions. Or, in this case, build unstoppable bots: Those best practice stacks gave genAI and machine learning bots a perfect testing ground of datasets and structures to learn from and reverse-engineer attacks.
We’ve seen this kind of tailored attack approach before. In one study we did of customer fraud attack data, we saw that many bot assaults included a sophisticated division of labor—100% of observed bot attacks were set up by human-testing beforehand. For example, with one customer we tracked how human fraudsters carefully probed fraud defenses, inputting dummy data in order to expose fraud controls. They then programmed bots to get around those specific controls and exploit any vulnerabilities. These were not indiscriminate mass-bot-attacks on any target; they were meticulously tailored to bypass specific customers’ unique control mechanisms.
Add genAI, and fourth-generation bots that can almost perfectly mimic human behavior, and you get a bot army that can be deployed at scale with customized orders for different fraud layers.
The Rise of Next-Gen Bots: Building Better Behavior For A New Era of Fraud
The journey of fraud bots began with simple, first-generation scripts that made basic requests to websites using limited IP addresses. These early bots were easily detected and blocked through straightforward measures like IP blocklisting and user-agent analysis. But as technology advanced, so did the bots.
Second-generation bots introduced headless browsers, maintaining cookies and executing JavaScript, which made them more capable and harder to detect. Third-generation bots took it a step further by simulating basic human interactions, such as mouse movements and keystrokes, though they still lacked human-like randomness.
The game changer was the emergence of today’s fourth-generation bots. Behavioral analytics has long been considered one of the most advanced defenses against fraud bots, and this generation focused on specifically reverse engineering user behavior patterns. Behavioral analytics was built to distinguish between genuine human users and automated bots (and then let you know if that human was risky or trustworthy). So, bots evolved to better replicate human behaviors. Fourth-generation bots exhibit advanced human-like interactions, such as moving the mouse in random patterns and changing user agents while rotating through thousands of IP addresses. They even employ “behavior hijacking,” recording real user interactions to closely mimic human behavior, making them nearly indistinguishable from actual users.
The New NeuroID Bot Signal Put to the Test
The rapid evolution from first-gen to today’s fourth-gen bots has already rendered many traditional fraud protection tools ineffective on their own. Without advanced updates, NeuroID behavioral analytics could have been the next solution to fall. Luckily, our engineers and data scientists were ready.
Let’s look at one of our behavioral data points: mousing. NeuroID data scientists developed algorithms that identify the difference between smoothing an already smooth trajectory vs. smoothing a wiggly, natural trajectory. With fourth-generation bots, navigation has evolved to be much more human-like. But still, unlike the typical erratic motion of humans, our data science team noticed subtle behaviors, which are discrete signs of a bot or script. There are still bot giveaways, if your behavioral solution is advanced enough to find them.
A somewhat sophisticated bot will run a script that looks the same as humans: slower typing speed, human-like mouse movements, and behaviorally accurate transitions. But more sophisticated bots begin to break that pattern, and run the bots differently each time. Fortunately, our analytics cover it all.
(Want a better look at how our signals’ have evolved, include a case study comparison of fourth-gen bots? Read our new report, Fighting the Future of Fraud: Understanding and Combating Next-Gen Bots)
Fraudsters are growing increasingly adept at bypassing traditional defenses, and bots are evolving to be more and more human-like. The new pace of fraud bot evolution—combined with a very low barrier of entry to fraud, thanks to genAI and “bots-as-a-service” software—represents a significant shift in the fraud landscape. But while bots are getting smarter, so are NeuroID defenses. By combining behavioral analytics with device and network intelligence, you can create a multi-layered defense that adapts as quickly as bots evolve.