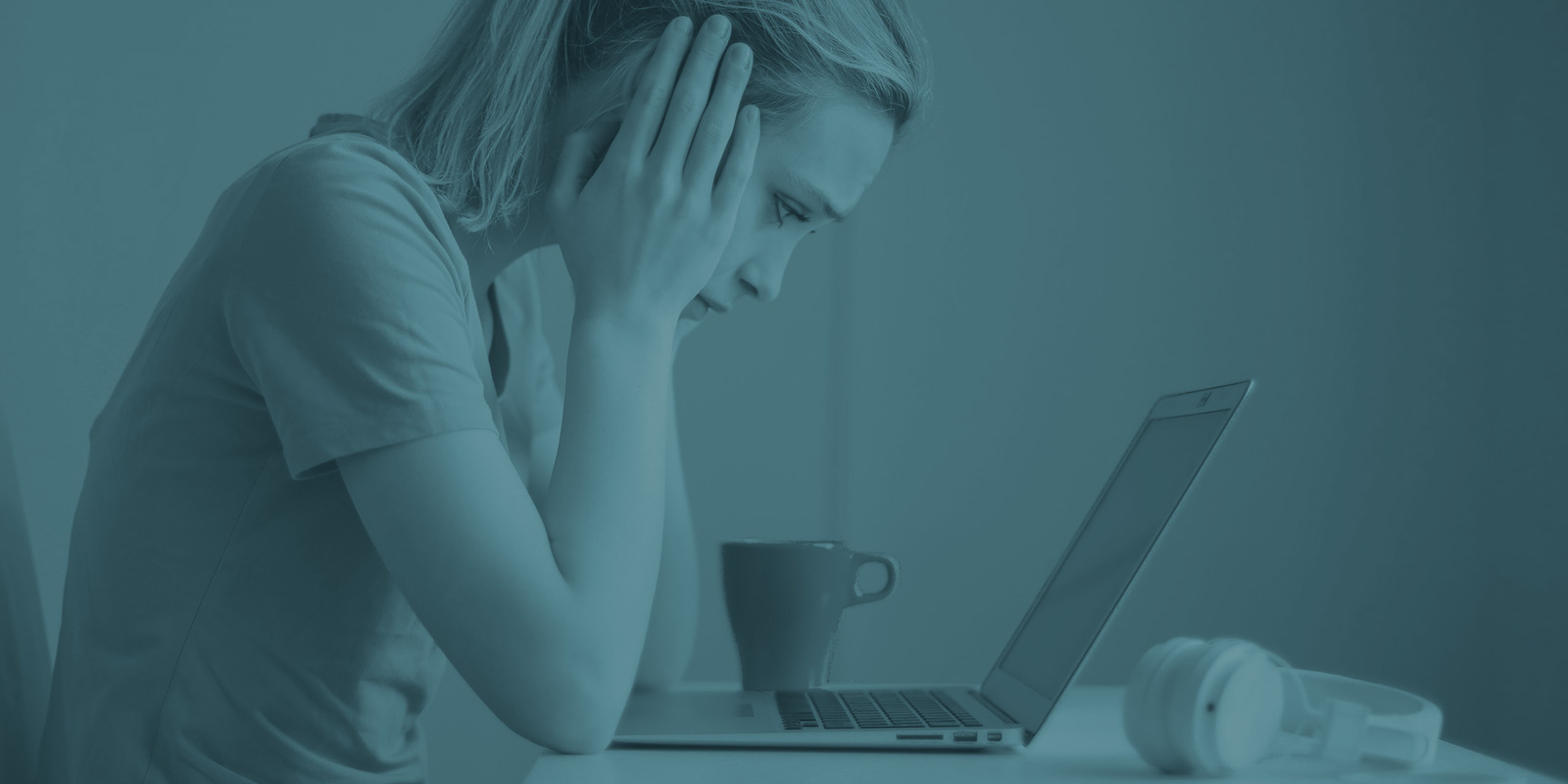
Stop Driving Away Good Customers: How to Reduce False Positives
Getting rejected never feels good – you know it, and I know it. Although in some instances rejection and failure can be translated into learning opportunities, some rejections mean lost revenue and customer trust. For top companies, overtooled digital fraud systems are doing just that, rejecting otherwise good customers in droves. As more and more business shifts online, false positives are a costly problem for companies embracing digital transformation.
False Positives Can Be Worse Than Fraud
As digital ‘first impressions’ overtake in person introductions, critical interactions between potential customers and businesses too often fall flat. Customers facing friction with an online purchase or application can easily choose a competitor just by clicking on the next search result.
Losing Customer Trust (And ROI)
Fraud false positives, when an innocent user is misidentified as a fraudster can lead to an immense loss in immediate revenue and in potential lifetime customer value. In fact, a study published by the Merchant Risk Council found that 33% of customers that are falsely declined, will never return to that site. Not to mention the immeasurable loss of customer trust and negative consumer perception as those same users share their experience with others.
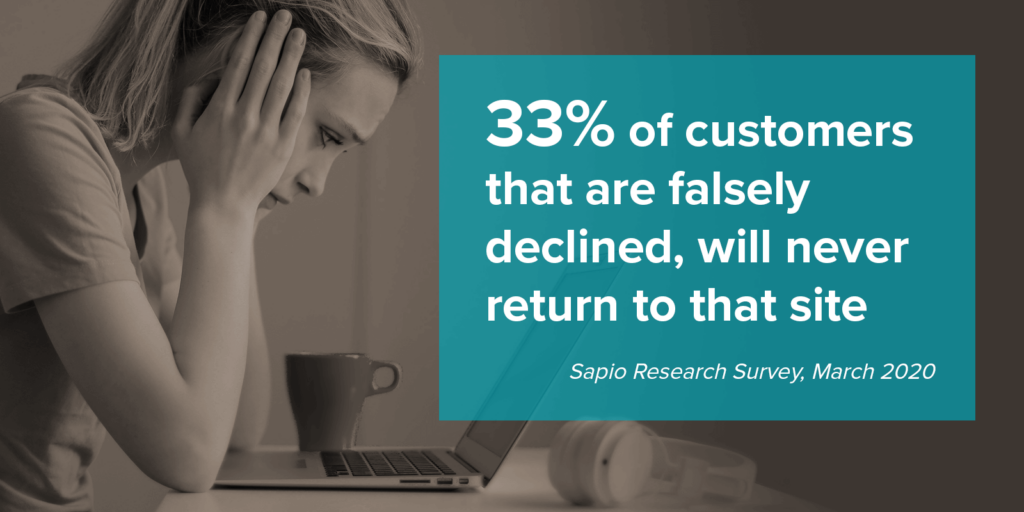
The report goes on to say:
…the average online store declines 2.6% of all orders because they suspect fraud. The higher the purchase price, the higher the percentage of declines. Merchants decline roughly 3.1% of orders over $100, for example.
You don’t need to be a mathematician to understand the magnitude of this systemic issue. The opportunity cost can’t be overstated.
The True Cost Of False Positives
Despite over a decade working with fraud teams, helping hundreds of companies fight fraud and reduce losses caused by cybercriminals, data breaches, and poor verification strategies –- what has been most alarming is just how much money businesses lose each year to false positives – the decline of good customers that, are flagged as suspicious and declined.
While combating fraud is an incredibly worthwhile endeavor, false positives are a more significant issue than most organizations realize. According to an Aite Group report on Balancing False Declines and Fraud Prevention, between 2017-2019, 62% of merchants reported their false positive rates had increased. This same report predicts that the losses will grow to $443 billion by 2021. This number dwarfs losses from fraudulent purchases.
All these numbers are staggering and highlight the weight and critical importance of how customer interactions are handled in a digital setting, as well as the importance of optimizing the user experience while still preventing fraudulent activity.
How To Reduce Your False Positive Rate
While these numbers may seem a herculean effort to overcome, if organizations had better intelligence on genuine vs fraudulent customers, that number would be a fraction of what it is. The good news is that technology now exists to help companies keep fraudulent activity down, while improving their false positive rate by detecting the signals that are displayed by genuine customers. All without completely revamping their current fraud detection systems.
Recognize User Intent With Behavioral Analytics
Part of the difficulties in determining fraudulent behavior vs buyer behavior lies in current fraud detection technologies. Most current fraud technologies rely on static, historical data which is easily compromised. They are not programmed to recognize user intent based on behavior which is so crucial in detecting fraudster vs customer.
This is where behavioral analytics comes into play. Behavioral data, often called “digital body language” is information derived from the digital interactions of an online user. When a prospective customer taps, types, or swipes information into an online form or application their behaviors create data.
Behavioral data provides indicators of the intentions and experience that the user leaves behind with every interaction. It can provide deep insights into what users’ true intentions are (legitimate or nefarious) as well as the experience they have during their customer journey (confusion, frustration, confidence, etc). For most organizations, this behavioral data goes unmonitored and therefore unused, but is key to improving customer experience while still preventing fraud.
Improve The Customer Experience While Preventing Fraud
This data has always existed but until new technologies were developed to capture it companies could not monitor or analyze it. Now that this technology exists, companies can leverage this digital body language data to determine if a user is a fraudster or a customer and reduce false positives. It’s the critical missing piece in a business’ fraud detection and prevention system.
Neuro-ID’s behavioral analytics solution can be used in conjunction with current fraud prevention platforms without needing to completely overhaul the system. This means that the ability to decrease false positives while still preventing fraud is easy to utilize and does not require long, drawn out implementation processes or learning curves.
User Intent As A Tool For Fighting False Declines
By understanding the intentions of each visitor, your business can unlock the ability to confidently reduce the false positive rate for any organization. Through monitoring and translating the real-time digital body language of a digital interaction, Neuro-ID reveals the intent and experience of every prospect and customer by providing deep insight into which are genuine and want to buy, and which ones are trying to cyber-shoplift. That distinction alone has the potential to save billions of dollars annually.
Let us show you how you can reduce your false positive rate and increase conversions by seeing and understanding your real, good customers – the ones that clearly deserve a best first impression.
Tap into your behavioral data
Learn about Neuro-ID’s Behavior-as-a-Service platform and how to gain actionable insight in 3 easy steps
Editor’s note: This blog was originally published March 17, 2021 and has been updated for accuracy and comprehensiveness.