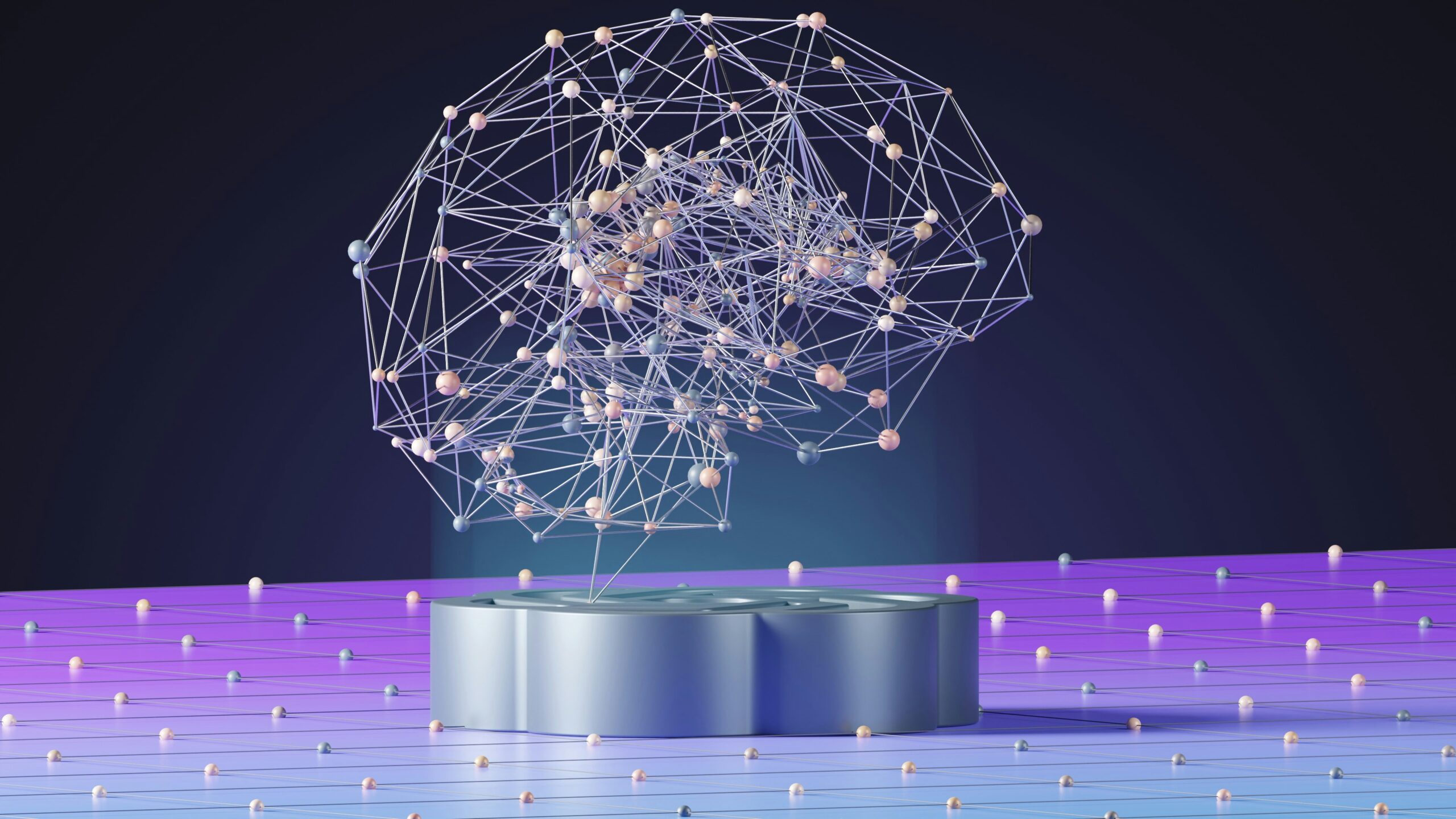
Who is Winning in the Race for GenAI Weaponization—Fraudsters or Fraud Teams?
Last month, I attended Fintech MeetUp 2024 and attended an AI-specific session with panelists from Google, NVIDA, Microsoft and the US Federal Reserve System. This group of industry giants discussed the many ways AI and genAI are set to revolutionize banking and fintech.
It was just one of the many genAI impact-analyses happening in industries all over the world. We know from our daily discussions with fraud professionals that genAI is constantly on their minds. Specifically, they’re worried that fraudsters may have already won the race, weaponizing genAI so quickly that the good guys will never be able to catch up.
How much of any genAI conversation is fear mongering and how much of it is foresight? During the Fintech MeetUp AI session, the panelists broke down some of the intimidating features of the AI revolution by focusing on the nuts and bolts of true AI integration.
Listening to the experts, I captured four major phases of genAI transformation. In each of these phases, financial institutions and fintechs are competing with fraudsters to gain the leg up—weaponizing AI for defense, like consumer data and revenue protection, or offense, like smarter and more proactive fraud detection. Let’s break genAI transformation down from the fraudster vs. fraud prevention side and see who’s gaining speed and who’s losing traction.
Phase 1: Leverage Copilots
Most businesses aren’t able to just start incorporating genAI right off the bat; they need to build up to it. Yogesh Agrawal, VP of Enterprise Al Business at NVIDIA, noted that genAI just isn’t accessible right away, “you have to start moderniz[ing] your data and computing infrastructure so that you can get to a stage where you can unlock the potential of AI and ask the right questions of your data.” So the panel advised starting with very specific problems and seeking out AI vendors to be your copilot. Leverage their expertise with plug-and-play solutions so you can start learning and modernizing.
Fraud Prevention
There are vendors and organizations entirely dedicated to using genAI to improve FIs and fintech workflows. However, few have the ability or focus on mitigation of AI-based fraud (NeuroID is one of them!).
Fraudsters
From genAI for human-like writing, voice authentication, and deep fake IDs, fraudsters are eager to share new ideas and provide support to one another on the dark web. Not only has the barrier to entry been dramatically lowered, but so has the cost. With FraudGPT costing only $1,700 for an annual subscription, it is one cheap copilot offering exponential rewards.
Winner: Fraudsters
For FIs and fintechs, prioritizing new vendor integrations and allocating costs takes time. Not to mention there are very limited options for fraud detection. Meanwhile, fraudsters have little to lose by trying new tools or following fraud guides on the dark web. They have the clear advantage in phase 1.
Phase 2: Build a High-Quality Data Foundation
The panelists then stressed the need to organize your data. Toby Brown, the Global Head of Banking Solutions at Google, noted that “80+% of data is unstructured. That’s honestly where a ton of insights is lost.” To capitalize on the power of AI, your data needs to be cleaned, organized, and properly tagged.
Fraud Prevention
There are two data foundation goals: build a profile for trustworthy user and a profile for a risky user. Profiles for trustworthy users can be developed from the first sign-up, attaching biometric, behavioral, device, and network data—in addition to their PII—to create an AI-enhanced pattern of that user. While there is still regulation and accuracy to work through, this area is well underway (and NeuroID is one of many vendors paving the way here).
For many organizations, fraud outcomes like defaults and chargebacks are well established. But more sophisticated fraud tagging around the type of fraud, like first-party or third-party, may be less detailed. But the more accurate and comprehensive fraudster profiles organizations build, the easier it may be to detect those fraudsters. Shifting your manual review team’s focus from just approving or denying actions to more sophisticated fraud investigation and tagging can help build this data library.
Fraudsters
For fraudsters, there is one data foundation goal: build a profile of a trustworthy user. With high-quality, accurate PII readily available for sale across the internet, fraudsters are more than equipped to feed cohesive and accurate data to their AI large language models (LLMs).
Winner: Fraudsters
With organizations still relying predominantly on PII for new accounts and transactions, fraudsters have the leg up. They can cut through KBAs, OTPs, and CAPTCHA (with a recent study proving bots solve CAPTCHA better and faster than humans), leaving FIs and fintechs in a sea of manual reviews. Again, fraudsters have the leg up.
Phase 3: Develop Computations for Building
Once the problem is outlined and the data foundation is in place, it’s time to build the genAI model. But for regulated organizations, this is no small undertaking. The good news, however, is fraudsters are also challenged by this, as it requires immense cooperation of data, strategy, and execution.
Fraud Prevention
Passing model risk governance (MRG) is the hardest challenge, according to the experts on stage at Fintech Meetup: “Working at a large incumbent bank, it would take 9-12 months on average to go through the process of figuring out what model you wanted to use and getting it into production,” said Toby. “Because you’d have to go through things like model risk management. You’d have to look at your policies around model risk. You’d have to figure out all this documentation. I think on average it’s something like 200 pages of documentation, for any given model.” And that’s for models where regulation is clearly defined. For AI, the waters are still uncertain making it even more difficult to align on what a safe and successful AI model looks like.
Fortunately, AI may be able to help explain itself. “You can have genAI explain what it’s doing and why and help you document [the decisions it’s making],” said Toby. There is still a human in the loop—often a MRG requirement.
Fraudsters
While the dark web offers copilots and PII in abundance, its strength is also its weakness—it’s decentralized. PWC collaborated with Stop Scams UK to research how AI technology impacts fraud and scams. So far, businesses haven’t reported AI attacks at scale (although it can admittedly be hard to know for sure if AI was involved). Scams and fake accounts are increasingly popping up, but fraudsters are prioritizing the easy wins first: organizations with weak or obvious controls that offer quick financial gain. Successful fraudsters don’t actually need AI to be profitable with so many technology laggards keeping low-tech fraud profitable. While more sophisticated attacks are expected soon, they’re not here yet.
Winner: Fraud Prevention
While all their data may not yet be organized, FIs and fintechs certainly have a leg up on the decentralized nature of fraudsters. While an argument may be made that fraudsters don’t need genAI models given the current success of their AI fraud vectors like deep fakes, synthetic ID creation, fake documents, and bots, these tools may not be enough to get through the AI model-focused defensive strategies for FIs and fintechs of the future. As smarter AI models are implemented and designed to detect AI-generated fraud, fraudsters will be forced to play catch up, developing smarter offensive techniques. And while regulators will provide checks and balances, they are also rooting for the good guys. “There are a lot of good foundational models, including open source models,” emphasized Sandeep Mangaraj, the Managing Director of Fintech for Microsoft. “So using the cloud, there is no reason why you cannot go and start experimenting, and not just experimenting, right? Like really start to operate, and then comes scaling.”
Phase 4: Scale & Transform
Today’s AI is generic. The next phase needs to be tailored specifically to the financial services industry. “Just like there is a language of biology, for which there are large language models, there is a language of financial services,” noted Yogesh, who sees this as the next wave of AI.
Fraud Prevention
Network effects are one of the largest resources available to FIs and fintechs. Banning their individual sizes with others in the space can create larger and more sophisticated models and databases. With the cost of mitigating fraud 4X higher than the actual fraud loss, there will be heavy incentives to continue scaling and modernizing fraud prevention. Not to mention the VC investments in AI for good are skyrocketing. But the final call will come down to how quickly the public and private sectors across countries learn and act.
Fraudsters
This transformative wave will expose more weaknesses. When banking went online, a host of weaknesses were revealed for FIs who didn’t realize all the vulnerabilities they had. As FIs and fintechs start to scale their use of AI, they will undoubtedly discover they have coverage gaps. Fraudsters will no doubt take advantage of these gaps, but they may not use large-scale LLMs to do so — more simple, low-tech offensive strategies may be enough.
Winner: Fraud Prevention
While there are still technology laggards, many FIs and fintechs have at least some modern fraud controls in their digital applications and self-serve workflows. There is a lot we don’t know about using AI at scale, “but a brave new world could be next where user interfaces fundamentally change and make it harder for fraudsters,” said Toby. That is to say, once an attack happens, it can be studied and better defended against, especially with AI learning and adapting. If governments, countries, and businesses can team up, this phase is theirs for the winning.
Ultimate Winner: Survival of the Most Prepared
In looking at the fraudsters and fraud prevention race across the phases, they seem tied, neck-in-neck towards a photo finish. And in fact, there probably won’t ever be a clear winner. It’s going to come down to survival of the most prepared. Fraud will always converge around the weakest links. For fraudsters, it’s about discovering new vulnerabilities and shaping new attack vectors. For fraud prevention, it’s going to be about staying agile through solutions that are predictive, real-time, and future-proof. NeuroID can help—talk to us to see how.